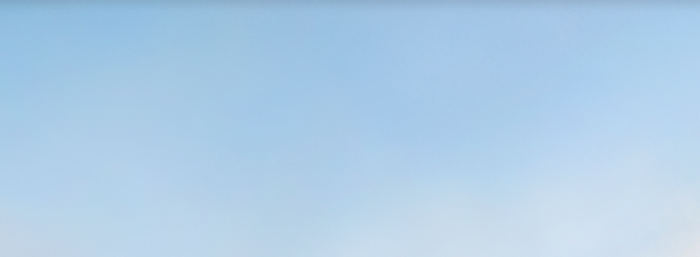
Sign up for the Newsletter
Join over 2,000 investors from the top hedge funds and long-only asset managers to receive a summary of the latest datasets, jobs, news, and events happening in the alternative data space.
What is Alternative Data?
Alternative data refers to data used by investors to evaluate a company or investment that is not within their traditional data sources (financial statements, SEC filings, management presentations, press releases, etc.). Alternative data helps investors get more accurate, faster, or more granular insights and metrics into company performance than traditional data sources. Over the last 10 years, increases in computing power and personal device usage created massive growth in data generation. As a direct outcome, a large number of companies emerged to collect, clean, analyze, and interpret data and provide it as a product that could inform investment decisions (“Alternative Data Providers”). See growth in alternative data providers selling to institutional investors in Figure 1.
Alternative Data Provider Stats
- Alternative Data Providers: 445
Alternative Data Use Growth
For funds to make use of these datasets for investment decisions, they have had to build out their data teams.
- The number of alternative data full-time employees (FTEs) at funds has grown ~450% in last 5 years.
- Most alternative data FTEs have 11+ years experience and do not have graduate degrees.
- Tech, Academia, and Data Providers are quickly becoming main channels for sourcing alternative data FTEs.
- Cost of an alternative data team starts at $1.5 – $2.5m.

Figure 2. Growth in funds with alternative data teams and full-time alternative data employees.
See our original Buy-side Alternative Data Employee Analysis for detailed breakdown of growth in alternative data team building on the Buy-side. Note: Updated methodology on the analysis cited above led to new estimate of 1,190 Data FTEs in 2017.
For most recent alternative data-related job posting at funds and providers, see the Jobs Page.
As funds have found use cases and applications for the increasing number of alternative datasets, their spend on alternative data has increased accordingly. (See Figure 3 – note: this includes spend on both datasets and infrastructure).

Figure 3. Buy-side spend on alternative datasets and infrastructure.
AlternativeData Stack
After thousands of conversations with investors, vendors, and experts, we have compiled the stack of top alternative data providers in the institutional investment space. The stack focuses on the top 100 data providers used by fundamental investors. It excludes market data, economic/macro data, and market news/industry publications.
Each provider’s position is intended to the firm’s product positioning relative to institutional investors. Data providers in the clusters towards the top are focused on data analysis and extracting insights from alternative data. Clusters that are positioned toward the bottom are more focused on data collection and quality assurance. and tend to not be directly consumed by fundamental analysts and PMs, but rather go through data brokers, the sell-side, or internal data teams for analysis.
For major players in alternative data providers broker out by data source and sector coverage, read on.
Major Types of Alternative Data
How is alternative data generated?
- Individuals: Social/Sentiment, Web Traffic, App Usage, Survey
- Business Processes: Credit/Debit Card, Web Data, Public Data, Email/Consumer Receipts
- Sensors: Geo-location, Satellite , Weather
- Data from business processes are typically more structured than data from individuals or sensors.
- Data cost: typically Business Processes > Sensors > Individuals.
What are the different categories of alternative data?
- App Usage – Data on app engagement and reviews. The level of data accuracy and usefulness depends on the app panel size, functions and features collected, and the level of user engagement. Popular use cases: gaming, food delivery, streaming services.
- Credit/Debit Card – Transaction data generated from credit and debit cards. This data is considered highly accurate when the transaction panel is large and covers a consistent user sample. Usually panels over 3 million consumers are considered large enough to be useful. These panels are some of the more expensive data licenses on the market. Popular use cases: Retail revenue tracking.
- Email/Consumer Receipts – Transaction data generated from email receipts. This data is accurate, but panels are typically smaller than credit/debit card panels and can be biased depending on the nature of the email receipt collection (often via an opt-in email or rewards app). Popular use cases: Retail revenue tracking.
- Geo-location – Foot traffic data available from WiFi signals (limited granularity and accuracy) or bluetooth beacons (higher accuracy, more expensive, less coverage). Popular use cases: Geography-specific retail foot traffic tracking.
- Public Data – Data from public resources. In its original form, this data is often difficult to access, not clean, not in a usable format (e.g. PDF). The value add of public data providers is the work of collecting, aggregating, and making the data actionable. Examples include SEC filings, patent data, government contracts, import/export data, etc. Popular use cases: patent data for tech company; supply chain imports for manufacturing; government contracts for construction company.
- Satellite – Data collected from satellites or (increasingly common) low-level drones. This data is expensive and of variable quality. Image processing is as important as data collection (raw data is not valuable to most investment teams). Satellite data on parking lots is only useful if a more direct measurement of store activity (geo-location data) or spend (credit card, email receipt) data is not available or beyond price range. Popular use cases: supply chain disruption tracking; agriculture yields tracking; construction tracking; oil & gas production/storage.
- Sell-side – Alternative data teams within large sell-side institutions. Combine new data and processing techniques with traditional sell-side research.
- Social/Sentiment – Data obtained from text processing of social media, news, management communications, and other sources. Sentiment data is relevant for some companies (think younger, more trading volume, more volatile) more than large, established corporations. The data is often more relevant to shorter-term traders as it does not always reflect fundamental business aspects. On the lower end of cost spectrum. Popular use cases: Event-driven sentiment tracking; Brand Virality/Advertising success.
- Survey – Data collected from surveys. This requires opt-in and panel diversity is variable depending on how good the provider is. This is a direct line in to consumer sentiment, rather than collecting it from text processing as in social/sentiment data. Popular use cases: brand preference; consumer behavior.
- Weather – Data on weather patterns collected from sensors. Popular use cases: agriculture and commodities.
- Web Data – Data scraped from public websites. This data comes in a wide range, from highly accurate and expensive to extremely raw and relatively inexpensive. This data is applicable where KPIs can be tracked by aggregating and analyzing large amounts of public-facing information, such as companies that publicize quantity sold and prices on each item page. This data can be extremely granular. Popular use cases: e-commerce; auto sales; airlines bookings; travel bookings; job postings.
- Web Traffic – Data on quantity, demographics, and history (clickstream) of users visiting a certain website. This is popular for tracking e-commerce efforts. Popular use cases: travel bookings; e-commerce.
- Other – There are many other popular datasets, including point-of-sale data, ad spend data, pricing data, and much more. These are not yet broad enough to capture a full section.
Which are the most popular datasets for investors?
- Data source with the greatest number of providers:Social/Sentiment
- Highest grossing data source: Credit/Debit Card
- Most utilized datasets: Web Data, Credit/Debit Card
- Most insightful datasets: Credit/Debit Card, Web Data
- Least insightful datasets: Geo-location, Satellite
Major Players in Alternative Data
By Sector:
- Autos
- China
- Consumer
- Energy
- Internet
- Transportation
- Travel
By Data Type:
- App Usage:
- Credit/Debit Card:
- Email/Consumer Receipts:
- Geo-location:
- Public Data:
- Satellite:
- Social/Sentiment:
- Survey:
- Weather:
- Web Data:
- Web Traffic:
Must Read
Buy-side Alternative Data Full Time Employee Analysis
We compiled a dataset of alternative data full-time employees (FTEs) on the buy-side to analyze the various recruiting trends impacting institutional investors. As competition for data talent heats up, it is essential to understand the landscape, background, and cost of these professionals.
Financial Times: Buy-side Alternative Data Spend
The Financial Times published an article titled “Asset managers double spending on new data on hunt for edge” featuring AlternativeData.org’s first analysis on buy-side industry spend on alternative data.
The Ultimate Guide to Selling Data to Hedge Funds
Hedge funds are a complex and opaque industry, but if you master the key players and product challenges, there’s no market in the world with a higher ASP and a faster sale cycle – the ingredients of a valuable subscription revenue business.
How to Integrate Investment Analysts, Data Analysts, and Engineers
How to build the team that can turn large inputs of alternative data into KPI estimates and company insights.
Takeaways from BattleFin Miami
Key takeaways from the meeting of 107 asset managers ($760bn AUM), 94 data providers, and ~100 other industry professionals in Miami from January 30-31.
AlternativeData.org Site Overview
Database
A cataloged database of all alternative data providers.
Jobs
Active listings of jobs at funds and data providers focused on the alternative data skillset.
News
A collection of all our news, articles, and newsletters documenting trend in the industry and educational resources on industry topics.